Thermalizer: Stable neural emulation of spatiotemporal chaos
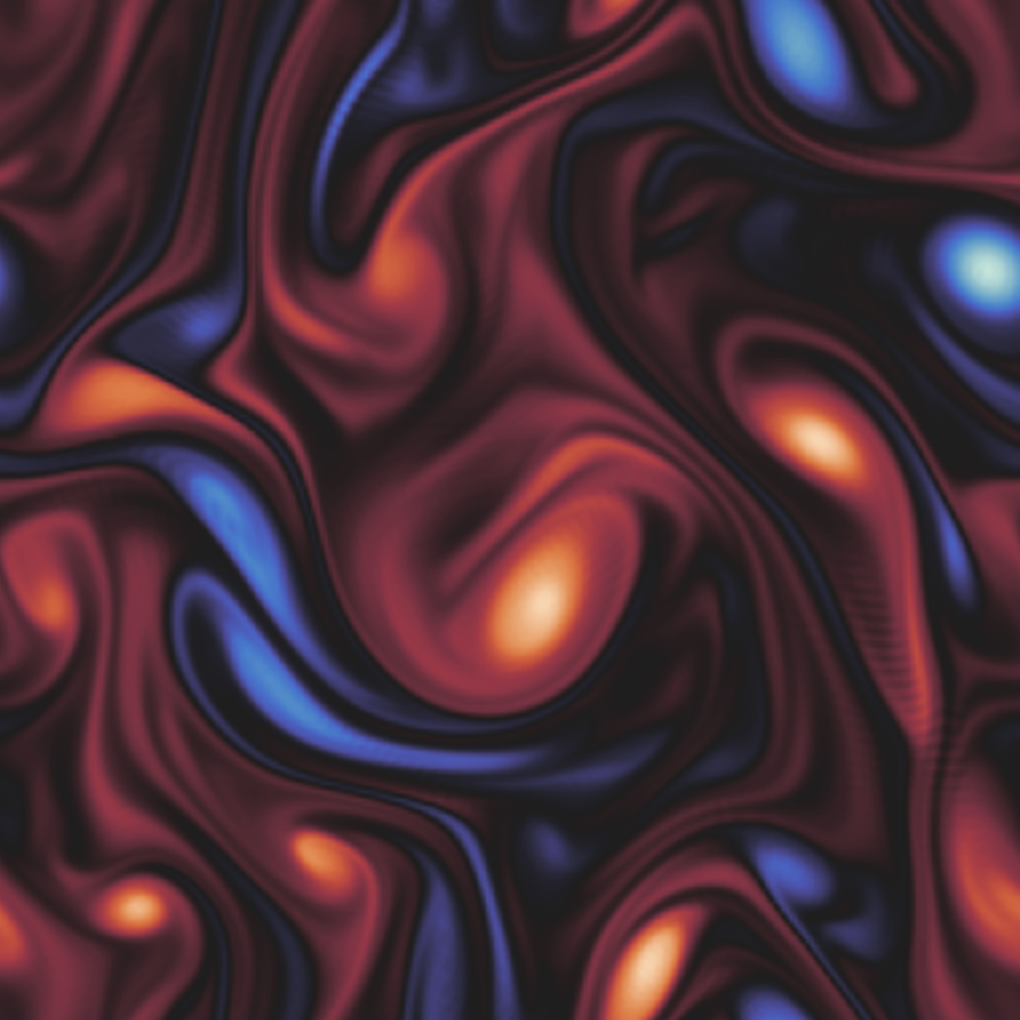
In this work we introduce the thermalizer, a modified diffusion model framework that can be used to stabilise autoregressive rollouts on the fly, extending the time-horizon of stable predictions by orders of magnitude.
Project ongoing...
Deep learning models of subgrid dynamics
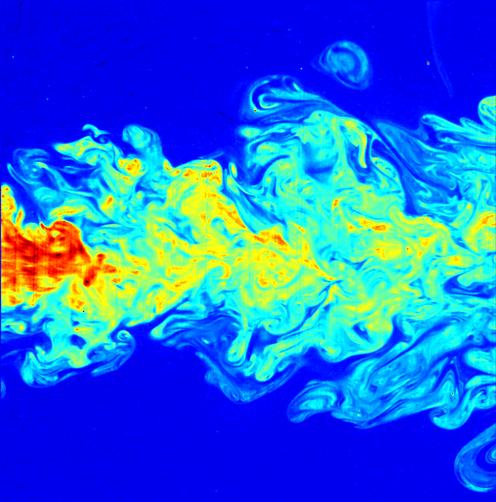
In this project we develop coupled physics + deep learning models of turbulent flows, where the machine learning component models the unresolved, subgrid dynamics, enabling accurate, coarse resolution simulations. In particular, we demonstrate how the stability of these coupled models can be improved by augmenting the training procedure with a neural network emulator.
Published at ICML 2023: Synergy of Scientific and Machine Learning Modeling Workshop
Cancer-net
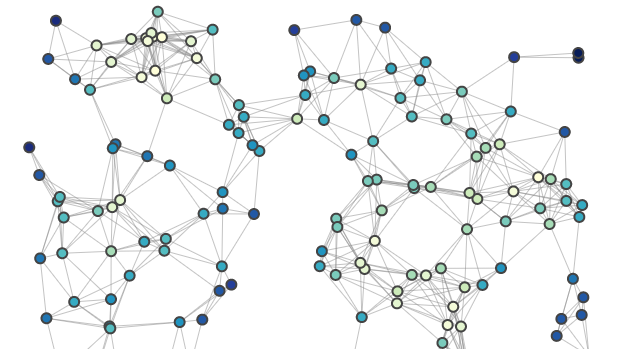
By grouping genes into subgraphs using biological prior knowledge, and using sparse connections between these graphs, we are building a binary classification model of prostate cancer tumors, which will predict whether or not a tumor is metaststic based on it's genetic markers. The motivation behind the graph network is to enable increased generalisability, and the sparse connections reduce the number of parameters of the model, enabling training on the small datasets available.
Submitted to Nature Machine Intelligence
Learnable wavelet neural networks for cosmological inference
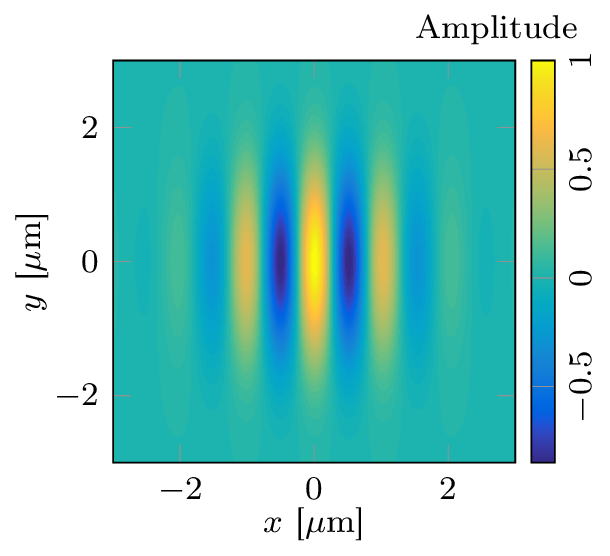
CNNs however require notoriously large amounts of data to train, and in the case of cosmological analysis, have to be trained on expensive numerical simulations. Given the limitations of computational resources, there is a strong motivation for designing models that can be trained on smaller amounts of training data. We designed a wavelet-based convolutional neural network, where the first 2 convolutional layers use wavelets instead of the traditional grid filters. The input fields are downsampled by factor of 2 after each convolution, meaning the wavelet layers are effectively performing an efficient compression of the data. The output of the wavelet convolutional layers are passed to a standard CNN of only 3 layers.
This model has an order of magnitude less learnable parameters than a standard CNN, and we show it dramatically outperforms a CNN at inference of cosmological parameters when in the regime of small training set sizes. Additionally, the wavelet filter parameters are included in the gradient descent, and can be used to understand where the information lies in the cosmological fields.
Published at ICML 2022 Machine learning for astronomy workshop
Compressing cosmological information
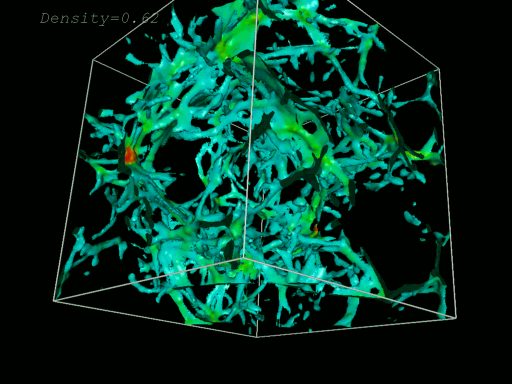
Whilst this information is extremely valuable, analysis of the Lyman-alpha forest is a highly complicated task, requiring expensive hydrodynamical simulations, and marginalisation over uncertain astrophysical effects which the absorption features we observe also depend on. Only a small number of groups have the expertise and scope required to perform this analysis. As a result of these obstacles, results from the Lyman-alpha forest are underutilised in the joint analysis of cosmological observations. In this work, we tackle this problem by showing that the cosmological information in the Lyman-alpha forest can be compressed into marginalised constraints on just two parameters, in a model-independent way and with negligible loss of information. This will enable groups to include these valuable measurements in their analysis, without having to runn their own simulations or perform their own marginalisation, and will dramatically boost the scientific impact of current and future Lyman-alpha forest observations.
Published in the Astrophysical Journal
Gaussian process emulator
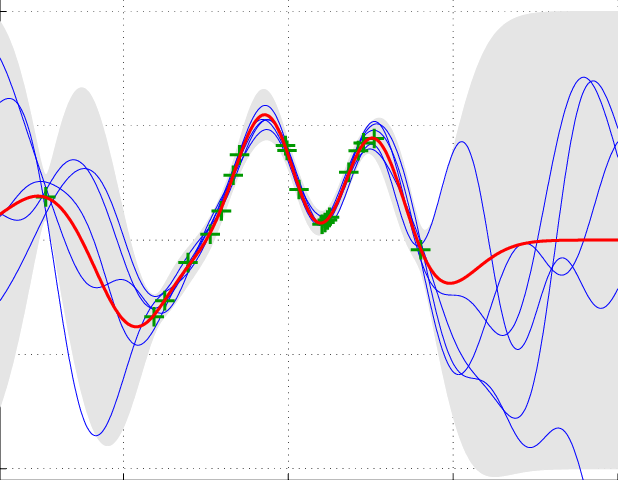
Published in JCAP
Gaussian process code
Neutrino mass degeneracy
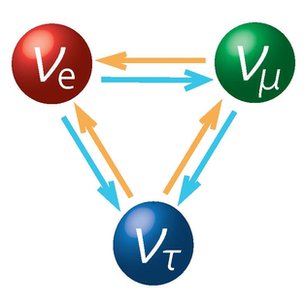
We find that the Lyman-alpha forest alone is unable to distinguish between the effects of neutrino mass and a uniform rescaling of the clustering amplitude. This has strong implications for the design of future modelling frameworks and likelihoods.
Published in JCAP
Precessing binary black holes
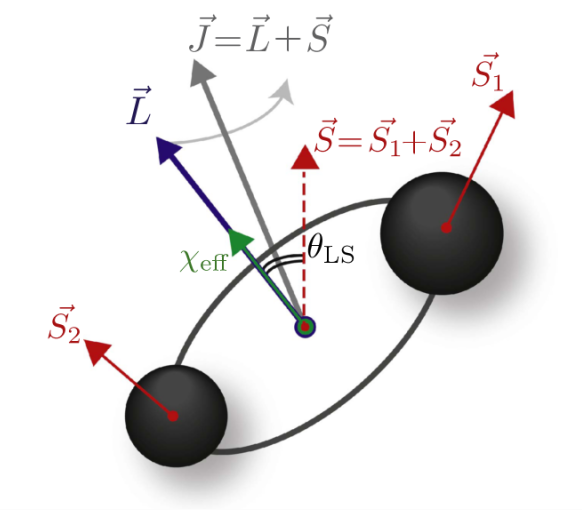
In this project I ran simulated mock merger events, and used Bayesian inference and Markov chain Monte Carlo analysis in order to understand our ability to observe this precession. I found that this strongly depends on the inclination of the binary system with respect to Earth, as well as it's location in the sky, due to parameter degeneracies.
Masters thesis - seed project for a publication in Physical Review D
Technical skills
Mathematical
- Linear algebra
- Probability & statistics
- Calculus
- Partial differential equations
Computational
- Python
- C++
- Linux/bash
- High performance/parallel computing
- git/github
- PyTorch, TensorFlow, Jax
Statistical/ML
- Bayesian Inference
- Markov chain Monte Carlo, Metropolis Hastings sampling
- Gaussian processes
- Linear regression, logistic regression
- Convolutional neural networks, vision transformers
- Generative modelling: VAE, diffusion models
- Graph neural networks/message passing networks
- Scattering transform